NIH Grant proposal for sale!
by bgood | Mar 21, 2014 | breast cancer, crowdsourcing, games, genegames, grant, gwaps, machine learning, NIH, r21, sulab, thecure |
Last November, Andrew and I submitted an R21 proposal for consideration by the NIH. Today, we received the summary statement. Since a lot of work went into writing it, I feel compelled to share it regardless of whether its ever funded (which currently seems like a longshot, but you never know). Perhaps someone will find a useful idea in there and the world will somehow be better for the work that we did. The summary statement is at the bottom – maybe that will also be useful to other folks thinking about begging for a living. The proposal involves ideas for extensions to the games and tools that, regardless of the lack of funding specifically for them, are slowly appearing at http://genegames.org/cure/ (thanks in part to the Google Summer Code).
(Note that the following is a resubmission. The introduction section is a response to the previous critiques and scores listed there in the table. The scores for this proposal are on the bottom of this post.)
Crowdsourcing Genomic Predictors of Disease Progression Using Serious Games
INTRODUCTION
This proposal sits at the interface between breast cancer, scientific knowledge, genomic data and community coordination. We hypothesize that data-driven attempts to make predictions of breast cancer prognosis can benefit from prior knowledge, and that current approaches for capturing knowledge from unstructured sources are inadequate. We suggest that, if properly coordinated, a motivated community could help address this challenge. In order to provide incentives and organization, we propose to create a “serious game”, also known as a “game with a purpose”. This game would serve as a focal point for community action oriented around understanding and predicting breast cancer prognosis, but could easily be generalized to other complex phenotypes. The game would attract the attention and focus the efforts of participants ranging from expert cancer biologists to students just learning about the field.
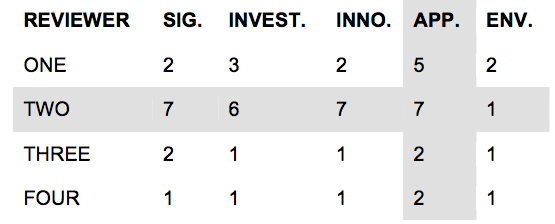 |
Table 1 (9-point rating scale (1 = exceptional; 9 = poor) ) |
This resubmission is a substantial rewrite of our original proposal. We made changes based on
additional preliminary data and the critiques offered by panel members. As summarized in Table 1, Reviewer 2 was the most critical reviewer, noting that our prototype game “lacked a playability factor”. This concern was cited as a primary weakness for all evaluation criteria except Environment. The three
other reviewers echoed this concern, as Approach was consistently judged to be the weakest area. The feedback from all four reviewers can be summarized as a need to significantly improve the game mechanics. They note, and we agree, that the game must be capable of attracting and holding the attention of a large audience. Therefore, we have made the following changes to our proposal.
- We have incorporated substantial new preliminary data. Despite the shortcomings of our current prototype, our preliminary data demonstrate that the proposed concept is fundamentally sound. In the twelve months since our original proposal was submitted, over 1,200 people independently discovered and collectively played 10,500 rounds of our prototype game. New players continue to register every week. This player population provides a key new resource, not available at the time the original proposal was submitted, for iteratively refining new game designs. We have adapted the proposal to clarify plans to apply a user-centered design strategy consisting of repeated cycles of evaluation with new and existing players followed by adaptations and further testing.
- Our plans for our full-length game are better described. Our prototype game was designed for a relatively narrow group of players with both substantial biological knowledge and a desire to play a casual game. Based on preliminary data and interviews with players, we altered our proposal to place a greater emphasis on stratifying the challenges in the game to better suit players with different degrees of expertise. In the current proposal, we added a significant new focus on providing a range of game levels to better meet the educational needs of beginners (see Specific Aim #1) and the tools to explore data desired by the most advanced player-scientists (see Specific Aim #2). We expect these changes to make substantial improvements in both the “fun factor” that the initial review perceived to be lacking and the value of the data collected from the more knowledgeable players.
- Our proposed budget now includes specific funds dedicated to consultants in game design (see Key Personnel).
- We have assembled an extensive network of colleagues with interest and expertise in scientific game development. Within this network, we have established agreements to work towards cross- pollination of our different player communities and to provide each other with invaluable discussions during early stages of development. (See letters of support from Stegman, Waldispuhl, Maclean, Himmelstein, and Khatib).
Aside from comments related to gamification, Reviewer 1 commented on a lack of clinical and translational expertise on our team, which we have addressed by recruiting additional support from colleagues at TSRI (See letters of support from Leyland-Jones, Salomon and Schork). The only additional comment received was encouragement from Reviewer 4 who said: “Definitely resubmit if this version does not receive funding.”
SPECIFIC AIMS
Breast cancer is the most common cancer in women. Molecular signatures for predicting prognosis and drug response could greatly improve the quality of care. Computational analyses of full genome expression datasets have indeed identified such signatures. However these signatures leave much to be desired in terms of their accuracy, reproducibility in validation studies and biological interpretability. Following similar trends in society, leaders of the research community have recently used crowdsourcing to focus the attention of many new data scientists on this problem through open competitions such as the Sage DREAM7 prediction challenge. While this very young approach has already yielded innovations, it has so far only been used to expand the search for and organize the work of datamining specialists. What is not known is how to expand the reach of crowdsourcing approaches aimed at identifying molecular signatures beyond data scientists to include other members of the scientific community and even of the general public. How can we recruit and organize people that can directly process the unstructured knowledge constantly accumulating in the literature to compose their own novel theories? How do we coordinate the efforts of experts, recruit and train students, and bring the minds of immunologists, developmental biologists, ecologists, economists, engineers, and interested citizen scientists to bear on this crucial problem?
Our long-term goal is to identify a collection of re-usable design patterns that leverage human knowledge and reasoning at the scale of the Web to improve the process of identifying molecular patterns associated with complex biological phenotypes. The overall objective of this proposal is to generate a better predictor of breast cancer prognosis. Our central hypothesis is that a scientific discovery game can capture knowledge and human reasoning that can be combined with existing machine learning methods to produce more effective predictors. We arrived at this hypothesis based on (1) recent successes in scientific crowdsourcing such as the DREAM challenges, (2) impressive results from similar games with a purpose such as Foldit, Fraxinus, and Phylo and (3) accumulating evidence of the value of prior knowledge in the discovery of complex predictive patterns in cancer. Further, we have already succeeded in attracting more than 1,200 players – hundreds of whom had postgraduate degrees – to play a simple prototype game (see Preliminary Data). When completed, the proposed expansions and improvements to this discovery-oriented game will allow us to collect an unprecedented database of manually-generated, hypothetical connections between molecular and clinical variables and breast cancer prognosis. This will offer the potential to create better predictors by providing machine learning methods with information not otherwise accessible. Perhaps equally important, this approach stands to greatly increase public engagement in and understanding of the challenges of modern “big data” biomedical science. We will achieve these goals through the following specific aims.
Aim #1: Attract large numbers of people with wide-ranging backgrounds to learn about and to join in the process of identifying signatures of breast cancer prognosis.
Working Hypothesis: A compelling, web-based game will incentivize, educate and focus the efforts of many citizens, scientists and citizen scientists.
Aim #2: Capture a large volume of structured expert knowledge linking genes and clinical variables with breast cancer prognosis
Working Hypothesis: Within the population that is attracted to a scientific discovery game, we will identify a sub-population of players that are either knowledgeable (e.g. cancer researchers) or are intelligent and dedicated enough to become knowledgeable (e.g. patient advocates). We can identify such expertise based on actions taken in the game and provide these special players with access to expert-level tools that will allow them to compose, test and share the hypotheses that we seek to collect.
This work will produce and validate a new process for organizing large communities of volunteer knowledge workers. Using this framework, which alone will be valuable as a reusable methodology, we expect to generate novel prognostic signatures with both good predictive performance and greater biological relevance than those that currently exist. These signatures will stand to improve the state of the art in breast cancer prognosis and thereby improve treatment efficacy. In addition, the framework can be re-used to develop predictive signatures of drug response and other complex phenotypes.
RESEARCH STRATEGY
Significance. Many studies attempt to use genomic information to predict progression and treatment response for cancers and other complex diseases. Such predictors are of interest because, if sufficiently accurate, they could be used to personalize therapy and to cast insights into the molecular underpinnings of disease. Despite extended and intense research in a variety of areas, there are few clinically useful genomic predictors. Of the few that exist, the Oncotype DX® predictor for breast cancer prognosis is among the most widely used [1]. However, its effective application is limited to ER-positive, lymph node-negative tumors, and research into the development of more accurate prognostic predictors across all the subtypes remains highly active [2]. As a case in point, in the summer of 2012, ten years after the first major attempts to produce genomic predictors of breast cancer prognosis [3], SAGE Bionetworks launched a large-scale public contest to spur research in this area because suitably accurate predictors still had not been found [4]. Though there has unquestionably been progress in the past decade, it has been incremental at best.
We suggest that a fundamentally new methodology is needed to make significant strides on this difficult problem. In this proposal, we introduce a new approach that taps into the massive reservoir of biological knowledge currently trapped in unstructured text and in the minds of scientists. Since 2000, more than 160,000 publications related to breast cancer have been added to PubMed (http://tinyurl.com/brsince2000). Our approach provides a new mechanism for marshaling this knowledge for the purpose of building better predictors. If successful, it will produce a new collection of more accurate, more interpretable predictors of breast cancer progression. These findings would be significant for the following reasons.
- More accurate prognoses can be used to more effectively personalize treatment.
- More interpretable predictors improve approval chances for clinical tests and inspire further research.
- Many similarly structured problems could be addressed using the proposed approach.
Innovation. While many variations exist, the standard paradigm for translating high throughput experimental data (e.g. whole genome RNA expression profiles) into predictors of disease progression follows this basic pattern: (1) assemble a discovery/training dataset, (2) rank attributes according to some univariate statistic, (3) filter all but the top N attributes arbitrarily, (4) select a classification algorithm, (5) evaluate performance in cross-validation experiments and on external test datasets. Emphasis is placed on single-dataset analysis and pre-existing biological knowledge is only considered post hoc. The predictors generated with this approach consistently have problems in secondary and tertiary validation studies and in the stability of the genes selected using different training datasets [5]. Recently, methods driven by structured prior knowledge in the form of protein-protein interaction networks [6, 7], pathway databases [8, 9] and information gathered from pan-cancer datasets [10, 11] have been introduced. These methods guide the search for predictive gene sets towards cohesive groups related to each other and to the predicted phenotype through biological mechanism. In doing so, they have improved the stability of the gene selection process and the biological relevance of the identified signatures. These techniques hint at the potential of strategies that marry a top- down approach based on established knowledge with a bottom-up approach based directly on experimental data, but they have not yet produced substantially greater accuracy than other approaches. We contend that this is due in part to the lack of relevant structured knowledge to compute with. The proposed research seeks to provide a new mechanism to rapidly and inexpensively capture targeted biological knowledge that can be used directly to improve the inference of genomic predictors. This innovative approach opens up access to knowledge not currently represented in any structured database and offers a high-throughput mechanism to apply human reasoning to the predictor inference challenge.
Preliminary Data. In Sept. 2012, we released a simple proof-of-concept game called ‘The Cure’ (http://genegames.org/cure/). In this game, 1,250 genes are randomly distributed (twice) into 100 game boards, each with 25 genes. On each board, the player competes with a computer opponent to select the highest scoring set of 5 genes (Fig. 1). Each player’s score is determined by using labeled training data to infer and test decision tree classifiers that predict 10-year survival using expression data from just the selected genes. The better the gene set performs in generating predictive decision trees, the higher the score. When the player defeats their opponent, they move on to play another board and multiple players play each board. Information from the Gene Ontology, RefSeq, Entrez Gene and PubMed is provided through the game interface (see black tabs in Fig. 1) to aid players in selecting their genes. Players are also free to make use of external knowledge sources.
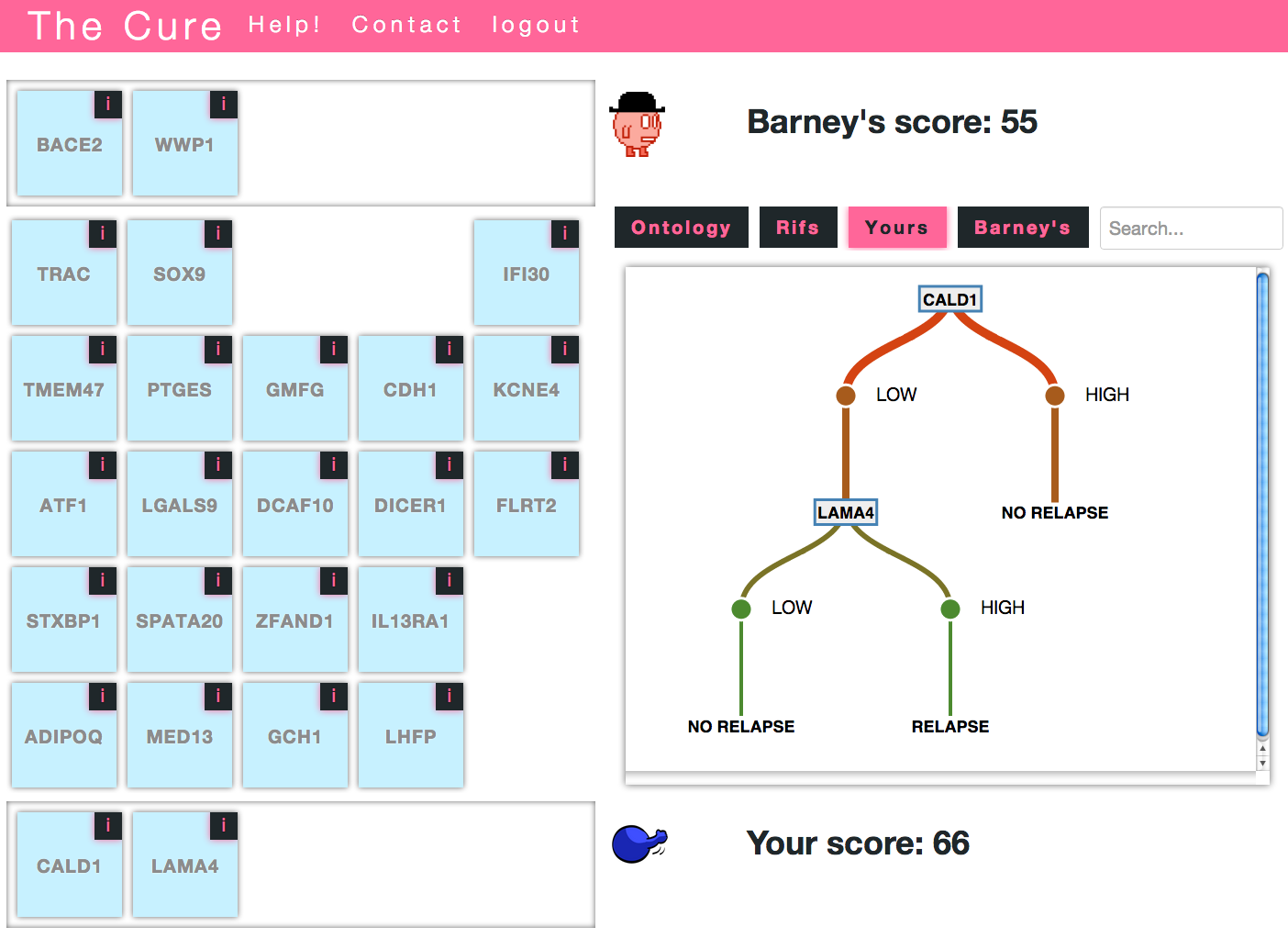 |
Figure 1. Prototype game. The player and the computer alternate in choosing genes from the board and adding them to their hand (bottom row and top row, respectively) until each player has selected five genes. The tabbed display at right provides hyperlinked information from the Gene Ontology and NCBI Gene RIFs and shows decision trees formed from the players’ selected genes. |
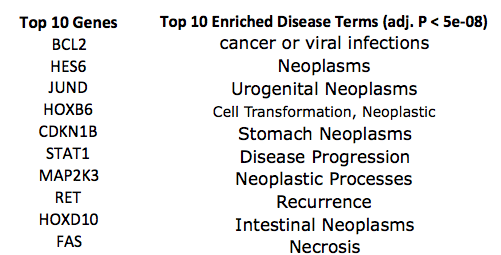 |
Figure 2. Top 10 genes and enriched disease terms linked to top 82 genes derived from game play data. |
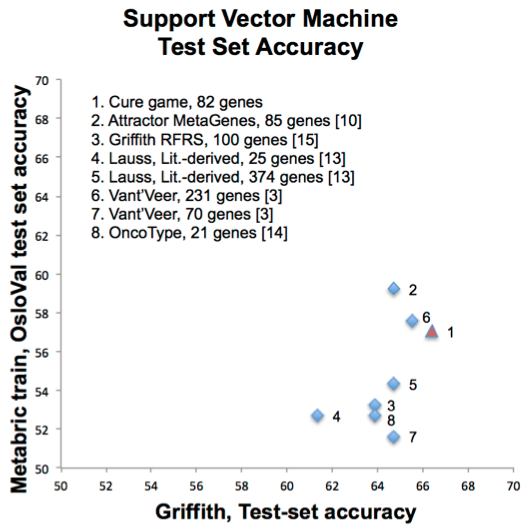 |
Figure 3. 10yr survival accuracy. SVMs trained using prior published gene sets and game-derived 82- gene set. X-axis Griffith 2013 train/test set [13], Y-Axis Oslo validation set with Metabric training set [4]. |
Between Sept. 7, 2012 and Oct. 28, 2013, 1,227 players registered and collectively played 10,549 games. 35% of the players reported completion of a post-graduate degree and 33% indicated expertise in cancer biology. Beyond initial announcements in Sept. 2012, we did not promote the game in any way, yet new player registrations have continued with the most per month (192) received in May, 2013.
We analyzed games from players with both a Ph.D. and knowledge of cancer and found a set of 82 genes chosen at frequencies above chance (p less than 0.001). Using disease-annotation enrichment analysis [12], we found that the 82 gene set was highly enriched for genes related to cancer (Fig. 2). We also compared the performance of classifiers trained using these 82 genes versus classifiers trained using prior published predictor genes (see [3, 10, 13-15]). The game-derived gene set resulted in comparable accuracy to prior gene sets in two large breast cancer profiling data sets (Fig. 3). While this simple prototype game did not produce a statistically significantly better classifier than prior approaches, these preliminary data did demonstrate that many knowledgeable people will play games oriented around breast cancer prognosis and that valuable information can be captured from the results of their play.
Specific Aim #1: Attract large numbers of people with wide-ranging backgrounds to learn about and to join in the process of identifying signatures of breast cancer prognosis.
Introduction. The value of any crowdsourcing initiative is directly proportionate to the number of participants. The more people that get involved, the more overall work that can be accomplished and the greater the chances of discovering exceptional individuals that can independently make important contributions. The objective of this aim is to produce a system capable of focusing the attention of thousands of people on the challenge of identifying prognostic molecular signatures for breast cancer. To attain this objective, we will test the working hypothesis that a compelling, Web-based game can incentivize, educate and direct the efforts of many citizens, scientists and citizen scientists. Our approach centers on applying a user- centered design process [16] to iteratively construct a game that appeals to a broad audience. There are two rationales for seeking a large, heterogeneous player population. 1. The more people we attract to the game overall, the more people with expert knowledge will be identified. 2. Non-expert players can contribute useful work based on their ability to read, their ability and desire to learn, and their innate ability to translate information into new hypotheses.
When the proposed studies have been completed, we anticipate having access to a very large population motivated to help in the process of understanding breast cancer and focused by the tasks defined in the game. The first expected product would be new ranked gene lists based on the knowledge and information processing abilities of this community as expressed through their actions in the game.
Justification and feasibility. Serious games or “games with a purpose” are a new form of crowdsourcing that explicitly employs fun as a primary incentive for volunteer participation [17]. These kinds of games have recently been used to help solve several complex biological problems. The most successful example is Foldit (http://fold.it), a game that has recruited more than 300,000 players and solved an impressive string of challenges in computational protein folding [18-20]. Of the Foldit players’ many achievements, perhaps the most notable is the design of a new protein folding algorithm with performance competitive to professionally- created solutions [21]. Another successful game, Phylo has attracted more than 12,000 players that have improved upon very large multiple sequence alignments [22], and recently Fraxinus has harnessed Facebook users to improve genome assembly [23]. Foldit, Fraxinus and Phylo have demonstrated that many people are interested in playing biological games and that these games can result in tangible contributions to research.
Research Design. The game that is the subject of this specific aim will provide an entertaining and educational experience that allows players to interact directly with genomic datasets related to breast cancer. Building on what was learned from the prototype, it will add the concept of levels and will introduce a number of new game mechanics to incentivize play. Before detailing the specific phases of planned work, we introduce some of the core concepts of the new design.

Levels. By stratifying the game into different levels of difficulty we will provide all players with games that are rewarding and fun. As players move up to higher levels, they will earn access to greater numbers of features (Fig. 4). In the first training stage, players will make simple choices, answering questions like “which factor is more useful for predicting prognosis, ethnicity or the number of lymph nodes infiltrated by cancer cells?”. As they answer questions correctly, they will move up to new levels with greater complexity. The prototype game depicted in Fig. 1 shows what a middle level might look like. In that case, players choose groups of 5 predictive genes from a set of 25. In the highest levels, players will have full access to all available clinical and genomic features. Through this progression we will seek to keep players in a positive cognitive state known as “flow” in which they experience a “feeling of complete and energized focus in an activity” [24] (Fig. 4).
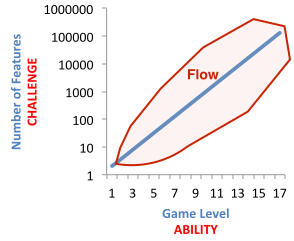 |
Figure 4. Designing game levels to keep players in state of flow. |
Incentives (“gamification”). In addition to the strong underling motivations to contribute to cancer research and to learn, the game will provide a variety of other compelling incentives for participation. Players will be able to earn status based on their position on leaderboards, feel a sense of accomplishment and discovery as they advance through levels, and bond with other members of the player community via discussion boards and chat functions. As in the prototype game, individual challenges will involve competitions with computerized opponents, but these competitions will also be enabled to occur directly between players.
Aggregation. Each of the choices made by players in these games can be considered a ‘vote’ for the selected feature (e.g. gene). While some votes will be random, their aggregation will reduce such noise and will reflect consensus knowledge in a unique, computable form (see Preliminary Data and [18]).
Planned activities. This work will be carried out in the following four phases.
Phase 1. Develop control questions. We will begin by working with experts in breast cancer genomics (see letters of support from Leyland-Jones and Griffith) to devise a set of questions covering the core elements of what is known in the field. These questions will be used in the early stages of the game to provide both educational material and a method for gauging player expertise.
Phase 2. Implement predictor evaluation service. The levels of the game created for this Specific Aim will focus on feature selection. In this phase, a web service will be constructed that accepts as input a set of features (e.g. the expression levels of genes) and responds with an empirical assessment of the value of that feature set. The service will incorporate multiple breast cancer datasets to improve the ability to assess predictor generalizability and will be provided via API. Initial datasets will include (at least) the 1,992 multi- subtype, multi-treatment samples from [25], the 858 untreated, ER+, LN-, samples from [15] and the 1,809 mixed samples from [26]. This API will be used by the game but will also be made available to the public. The datasets used in the server will be divided into training sets used to provide players with feedback and separate test sets used for subsequent evaluations.
Phase 3. Iterative Design. The new game will be developed in an iterative cycle of user-centered design. Game designs will be tested both in the existing player population on the Web (see Preliminary Data) and in small groups composed of local colleagues, students, and collaborating cancer experts. Small local groups will allow us to test ideas using mockups before implementing them and to maximize our understanding of individual player reactions [27]. Web-based experiments will allow us to measure progress at the community level in terms of metrics such as virality (n new players), stickiness (time spent on the game) and production (amount and quality of information collected). Critically, we will adopt an agile development strategy focused on quickly adapting game designs based on periodic (e.g. bi-weekly) in-person and community-level evaluations.
Phase 4. Promotion. When the game meets our expectations for playability and knowledge acquisition, we will use a variety of resources at our disposal to attract players. In particular, we will leverage the BioGPS website (viewed by more than 125,000 unique visitors annually) to solicit players via postings on the home page and emails to the more than 5,000 registered users [28]. In addition, leaders of several other scientific gaming initiatives have agreed to collaboratively promote all of our games (See letters of support from Waldispuhl, MacLean, Stegman, Himmelstein, Khatib). Finally, we are partnering with educational initiatives to promote the game’s use in massively open online courses (see letter of support from Taly).
Expected Outcomes. When the proposed studies for this aim have been completed, we expect to have produced a Web-based game oriented around the challenge of breast cancer prognosis that appeals to players ranging from novices to experts. This game will be both a useful educational tool and an effective mechanism to capture knowledge. Using the data captured from game play, we expect to produce a novel, knowledge- driven ranking of genes and clinical features with respect to their value for predicting breast cancer prognosis. This ranking will improve upon the ranking generated in the preliminary study based on the opportunity to collect substantially more data and on a far more refined approach for filtering the data based on player expertise made possible by the new, early stage levels.
This community-generated, ranked list will provide a useful source of prior knowledge for selecting features for classifier construction.
Potential problems and alternative strategies. A clear danger in any crowdsourcing initiative is the mentality that ‘if you build it, they will come’. If no one ends up playing this game, the value from this project will be minimal. However, based on our success in attracting more than 1,000 players to a single-level, unadvertised, hastily composed skeleton of the proposed game, we feel strongly that this event is very unlikely. If the game does not garner a sufficiently large audience (which we would assess based on the total amount of knowledge captured by the system within the first 2 months after the launch), we would shift our model by deploying the system in different contexts. Because the system will be developed for the Web, it would be easy to change the deployment from a standalone Web application into e.g. a Facebook game like Fraxinus [23] or a mobile application. We could also shift the game to focus more deeply on either the educational needs of students or on the needs of small communities of experts. Finally, the infrastructure for the game will be agnostic to the specific datasets being used and thus it will be possible to use the game for other biomedical challenges such as predicting organ transplant rejection (see letter of support from Salomon).
Specific Aim #2: Capture a large volume of structured expert knowledge linking genes and clinical variables with breast cancer prognosis
Introduction. Crowdsourcing discussions often focus on the “Long Tail” of small-scale contributors, which is the large number of contributors who add individually small (but collectively large) amounts of value. Yet the ecosystem of any successful system also contains a complementary contributor pool (the “Short Head”) that is comprised of a few key community members that individually produce a large quantity of high quality work. These individuals also motivate and guide the other contributors. The objective of this aim is to maximize the contributions from the experts in the player community. We will test the working hypothesis that experts will gain both professional value and enjoyment from the use of a tool, embedded in the sociotechnical context of a scientific discovery game, that will allow them to rapidly test their own hypotheses regarding connections between combinations of molecular features, clinical variables and breast cancer prognosis. Successful completion of this aim will (1) provide the research community with a tool that makes it easy to test complex hypotheses without the need to write programs and (2) enable the construction of a new kind of classifier that integrates knowledge spread across many hypotheses. These achievements would be important because they would increase the possibility of individual experts identifying novel signatures and because they would make it possible to create an ensemble classifier likely to outperform any independent signature. At a high level, the work proposed in this aim will reduce barriers to information flow between scientific communities by increasing the accessibility of big data for non-data scientists and increasing data scientist’s access to structured expert knowledge (see letter of support from Margolin).
Justification and feasibility. We propose to capture hypotheses structured as decision trees. For example, “if AURKA expression is high and TOP2A expression is low, then risk of recurrence is low”. This, and many more complex hypotheses, can be expressed as decision trees and tested automatically using available datasets. Decision trees provide a familiar, visual way to represent complex logical functions that may span many features and are the representation of choice for communicating complex diagnoses and treatment regimens among the medical community. They can be induced from data automatically [29], designed by experts or constructed using hybrid systems. Research has shown that involving people in the process of inferring decision trees can improve their predictive performance, decrease their size and increase their explanatory power [30-32]. The central technical product of the proposed research is envisioned to be a Web-based interactive decision tree builder.
Interviews with several cancer biologists that interacted with the prototype game exposed a consistent desire for greater control over the process of building the trees shown in the game (see letters of support from Griffith and Morin). Motivated by these interviews, we developed a prototype Web interface for building decision tree classifiers (Fig. 5). This proof of concept established the feasibility of the proposed research in our hands and has met with an enthusiastic response from early testers.
Figure 5. Prototype Decision Tree Builder. This tool allows search, selection and placement of features as split nodes as well as real-time performance evaluations on training data.
Research Design. The goals of this specific aim will be achieved in the following 4 phases.
Phase 1. Gather tree-building requirements and evaluation criteria. During this phase, we will
work directly with experts in breast cancer to manually define decision trees that reflect their current thought processes for linking clinical and molecular data to prognosis (see letters of support from Griffith and Leyland- Jones). This work will overlap with the first phase of Specific Aim #1 in that these trees will capture knowledge that we can use to train and evaluate all players. In addition, they will provide clear guidance as to the parameters that our proposed tree-building interface must be capable of representing. We will also use this phase to incorporate services for evaluating the hypotheses represented by these trees into the evaluation service described in Specific Aim #1, Phase 2. Working closely with clinical and translational experts at this stage will ensure that our evaluations are appropriate, for example that the chosen datasets accurately reflect relevant patient populations, and will set a solid foundation for the rest of the proposed research.
Phase 2. Tree-building tool development. This phase will focus on iterative design, implementation and testing of a Web-based tool for constructing and evaluating decision trees. The tool will be evaluated based on the ability of users to reproduce the expert-derived trees captured in Phase 1 and on their comprehension of the evaluations displayed by the system. The interface will allow users to search through features to use in split nodes within their trees. Individual features will include both clinical parameters (e.g. lymph node status) and molecular measurements (e.g. gene expression). In addition, meta-features that integrate signal from multiple smaller features such as the genomic instability index [33] or the aggregate expression of pathways, will be available as independent split nodes. For example, the system would allow users to test the hypothesis that low levels of BCL2 expression are predictive of poor outcome when tumors also have a high level of genomic instability. Coupled with the evaluation server mentioned in Aim 1:Phase 2, this tool will allow all scientists to use these high-throughput datasets to test the accuracy of their own biological models, a task that would be impossible without significant bioinformatics expertise.
Phase 3. Gamification. The code created in Phase 2 will be used to build the upper levels of the game described in Specific Aim #1. The game will provide incentives for use of the application and a rich social context for knowledge transfer among the player community. Players will be encouraged to share the trees that they create and to explain how and why they composed them the way they did. The results produced by the evaluations (e.g. the percent correct on training data), will be used to generate scores for a leaderboard. To reduce overfitting, scores will reward both high accuracy and low complexity. Following the pattern outlined in Aim #1, level progression in the tree-building stages of the game will orient around increasing the number of variables and levels of control available to the players.
Phase 4. Aggregation. All of the hypotheses of the user community, represented as decision trees, along with detailed information about each user’s actions taken in the game will be captured. Using this data, we will compose ensemble classifiers, akin to random forests [34]. In effect, individual players will act as highly intelligent components of a larger machine learning system. At a simple level, each of the trees submitted by users of the tree-building tool can be used as independent members of a decision forest. To make a prediction, each tree in the forest gets one vote and the class with the most votes is selected. During this phase of research, we will work to optimize such ensembles by (1) filtering out data from players with low levels of expertise (2) tuning the system to ensure adequate quality and diversity among the trees.
Expected Outcomes. Upon completion of the work proposed in this Aim, we expect to produce (1) a valuable piece of open-source, Web-based software for constructing and evaluating decision trees using biomedical data, (2) a community hub where researchers, citizen scientists and gamers can interact and explore new hypotheses relating molecular and clinical variables to breast cancer prognosis, (3) a large database of hypotheses structured as decision trees along with information about the level of expertise of their creators, and (4) ensemble classifiers that integrate the information collected from the community. The individual hypotheses and their aggregates into ensembles will provide an excellent opportunity to advance the state of the art in predicting breast cancer prognosis.
Potential problems and alternative strategies. Despite our preliminary data indicating the contrary (e.g. see letters of support from Taly, Griffith, and Morin), one potential problem may be a difficulty in attracting experts to the tree-building tool. While our main focus will be on the deployment of this tool within the game, an alternative strategy would be to refocus our effort on the development of a public, scientist-focused website like http://kmplot.com/. Following this strategy we would also allow users to keep uploaded trees and datasets private and thus reduce potential fears of “scooping”. Another problem may be that, even with the substantial human effort that could be captured by this system, the accuracy of predictors may not improve significantly. While our initial focus will be on identifying predictors that do improve on accuracy, an alternative is to re-align the game to provide greater rewards for constructing explanations of predictors that tie them closely to biological mechanism.
FUTURE DIRECTIONS.
Our high-level goal is to harness the power of the Web to help meet the challenges of the genome era. If successful, there are several natural extensions to this work that we will pursue. First, we will add the capacity for scientists to upload their own datasets and to expand the number of datatypes represented in the game framework (see letter of support from Morin). Second, we will expand the ecosystem of tasks that players can accomplish as part of these games. For example, we are already exploring mechanisms through which players can help to translate text from scientific articles into structures such as concept networks that could be used for predictor inference (e.g. see [6]). Third, we will explore other approaches from the domain of visual analytics that can be incorporated into our interface. Emerging techniques that, for example, enable users to visualize complex decision boundaries created by machine learning systems (e.g. [35, 36]) may help less experienced users make more important contributions.

TIMELINE
This proposal will require funding for two years. The work to achieve both specific aims will be done in parallel. It will begin with a period of high engagement with breast cancer experts to capture their knowledge for use in player evaluations and training in the lower levels of the game and to
establish requirements for the tree-building interface. We will also use this period to develop the
evaluation server that will be used to drive the games and assess performance. Next we will focus on the iterative development of games described in Aim #1 and the tree-building interface described in Aim #2. When the implementation of all of the levels of the game has reached a stable state we will focus on promotion. This work will conclude with an evaluation of all of the data collected, culminating with the assessment of the predictive quality of the ensemble predictor described in Aim #2.
BIBLIOGRAPHY & REFERENCES CITED
- Ross JS, Hatzis C, Symmans WF, Pusztai L, Hortobagyi GN. Commercialized multigene predictors of clinical outcome for breast cancer. Oncologist. 2008;13(5):477-93.
- Weigelt B, Pusztai L, Ashworth A, Reis-Filho JS. Challenges translating breast cancer gene signatures into the clinic. Nature reviews Clinical oncology. 2012;9(1):58-64.
- van ‘t Veer LJ, Dai H, van de Vijver MJ, He YD, Hart AA, Mao M, Peterse HL, van der Kooy K, Marton MJ, Witteveen AT, Schreiber GJ, Kerkhoven RM, Roberts C, Linsley PS, Bernards R, Friend SH. Gene expression profiling predicts clinical outcome of breast cancer. Nature. 2002;415(6871):530-6.
- Margolin AA, Bilal E, Huang E, Norman TC, Ottestad L, Mecham BH, Sauerwine B, Kellen MR, Mangravite LM, Furia MD, Vollan HK, Rueda OM, Guinney J, Deflaux NA, Hoff B, Schildwachter X, Russnes HG, Park D, Vang VO, et al. Systematic analysis of challenge-driven improvements in molecular prognostic models for breast cancer. Sci Transl Med. 2013;5(181):181re1.
- Xu JZ, Wong CW. Hunting for robust gene signature from cancer profiling data: sources of variability, different interpretations, and recent methodological developments. Cancer Lett. 2010;296(1):9-16.
- Dutkowski J, Ideker T. Protein networks as logic functions in development and cancer. PLoS Computational Biology. 2011;7(9):e1002180-e. (PMC3182870)
- Winter C, Kristiansen G, Kersting S, Roy J, Aust D, Knösel T, Rümmele P, Jahnke B, Hentrich V, Rückert F, Niedergethmann M, Weichert W, Bahra M, Schlitt H, Settmacher U, Friess H, Büchler M, Saeger H-D, Schroeder M, et al. Google goes cancer: improving outcome prediction for cancer patients by network-based ranking of marker genes. PLoS computational biology. 2012;8(5):e1002511-e.
- Bild A, Yao G, Chang J, Wang Q, Potti A, Chasse D, Joshi M-B, Harpole D, Lancaster J, Berchuck A, Olson J, Marks J, Dressman H, West M, Nevins J. Oncogenic pathway signatures in human cancers as a guide to targeted therapies. Nature. 2006;439(7074):353-7.
- Su J, Yoon B-J, Dougherty E. Accurate and reliable cancer classification based on probabilistic inference of pathway activity. PloS one. 2009;4(12):e8161-e.
- Cheng WY, Ou Yang TH, Anastassiou D. Development of a prognostic model for breast cancer survival in an open challenge environment. Sci Transl Med. 2013;5(181):181ra50.
- Cheng WY, Ou Yang TH, Anastassiou D. Biomolecular events in cancer revealed by attractor metagenes. PLoS Comput Biol. 2013;9(2):e1002920. (PMC3581797)
- Wang J, Duncan D, Shi Z, Zhang B. WEB-based GEne SeT AnaLysis Toolkit (WebGestalt): update 2013. Nucleic Acids Res. 2013;41(Web Server issue):W77-83. (PMC3692109)
- Lauss M, Kriegner A, Vierlinger K, Visne I, Yildiz A, Dilaveroglu E, Noehammer C. Consensus genes of the literature to predict breast cancer recurrence. Breast Cancer Res Treat. 2008;110(2):235-44.
- Paik S. Development and clinical utility of a 21-gene recurrence score prognostic assay in patients with early breast cancer treated with tamoxifen. Oncologist. 2007;12(6):631-5.
- Griffith O, Pepin F, Enache O, Heiser L, Collisson E, Spellman P, Gray J. A robust prognostic signature for hormone-positive node-negative breast cancer. Genome Medicine. 2013;5(10):92.
- Gould J, Lewis C. Designing for usability: key principles and what designers think. Commun ACM. 1985;28(3):300-11.
- von Ahn L, Dabbish L. Designing games with a purpose. Commun ACM. 2008;51(8):58-67.
- Good B, Su A. Crowdsourcing for bioinformatics. Bioinformatics. 2013;29(16):1925-33.
- Cooper S, Khatib F, Treuille A, Barbero J, Lee J, Beenen M, Leaver-Fay A, Baker D, Popovic Z, Players F.
Predicting protein structures with a multiplayer online game. Nature. 2010;466(7307):756-60.
(PMC2956414)
- Khatib F, DiMaio F, Cooper S, Kazmierczyk M, Gilski M, Krzywda S, Zabranska H, Pichova I, Thompson J,
Popovic Z, Jaskolski M, Baker D. Crystal structure of a monomeric retroviral protease solved by
protein folding game players. Nat Struct Mol Biol. 2011;18(10):1175-7.
- Khatib F, Cooper S, Tyka MD, Xu K, Makedon I, Popovic Z, Baker D, Players F. Algorithm discovery by
protein folding game players. Proceedings of the National Academy of Sciences of the United States of
America. 2011;108(47):18949-53. (PMC3223433)
- Kawrykow A, Roumanis G, Kam A, Kwak D, Leung C, Wu C, Zarour E, Sarmenta L, Blanchette M,
Waldispuhl J. Phylo: a citizen science approach for improving multiple sequence alignment. PloS one. 2012;7(3):e31362. (PMC3296692)
- MacLean D. Changing the rules of the game. eLife. 2013;2.
- Chen J. Flow in games (and everything else). Commun ACM. 2007;50(4):31-4.
- Curtis C, Shah SP, Chin SF, Turashvili G, Rueda OM, Dunning MJ, Speed D, Lynch AG, Samarajiwa S,
Yuan Y, Graf S, Ha G, Haffari G, Bashashati A, Russell R, McKinney S, Group M, Langerod A, Green A, et al. The genomic and transcriptomic architecture of 2,000 breast tumours reveals novel subgroups. Nature. 2012;486(7403):346-52. (PMC3440846)
- Gyorffy B, Lanczky A, Eklund AC, Denkert C, Budczies J, Li Q, Szallasi Z. An online survival analysis tool to rapidly assess the effect of 22,277 genes on breast cancer prognosis using microarray data of 1,809 patients. Breast Cancer Res Treat. 2010;123(3):725-31.
- Barrington L, Turnbull D, Lanckriet G. Game-powered machine learning. Proceedings of the National Academy of Sciences. 2012;109(17):6411-6.
- Wu C, Macleod I, Su AI. BioGPS and MyGene.info: organizing online, gene-centric information. Nucleic Acids Res. 2013;41(Database issue):D561-5. (PMC3531157)
- Quinlan JR. Induction of Decision Trees. Machine Learning. 1986;1(1):81-106.
- Mihael A, Christian E, Martin E, Hans-Peter K. Visual classification: an interactive approach to
decision tree construction. Proceedings of the fifth ACM SIGKDD international conference on
Knowledge discovery and data mining; San Diego, California, USA: ACM; 1999.
- Malcolm W, Eibe F, Geoffrey H, Mark H, Ian HW. Interactive machine learning: letting users build
classifiers. Int J Hum-Comput Stud. 2002;56(3):281-92.
- van den Elzen S, van Wijk JJ, editors. BaobabView: Interactive construction and analysis of decision
trees. Visual Analytics Science and Technology (VAST), 2011 IEEE Conference on; 2011: IEEE.
- Bilal E, Dutkowski J, Guinney J, Jang IS, Logsdon BA, Pandey G, Sauerwine BA, Shimoni Y, Moen Vollan
HK, Mecham BH, Rueda OM, Tost J, Curtis C, Alvarez MJ, Kristensen VN, Aparicio S, Borresen-Dale AL, Caldas C, Califano A, et al. Improving breast cancer survival analysis through competition-based multidimensional modeling. PLoS Comput Biol. 2013;9(5):e1003047. (PMC3649990)
- Breiman L. Random Forests. Machine Learning. 2001;45(1):5-32.
- Migut M, Worring M, editors. Visual exploration of classification models for risk assessment. Visual
Analytics Science and Technology (VAST), 2010 IEEE Symposium on; 2010 25-26 Oct. 2010.
- Poulet F. Towards Effective Visual Data Mining with Cooperative Approaches. In: Simoff S, Böhlen M,
Mazeika A, editors. Visual Data Mining: Springer Berlin Heidelberg; 2008. p. 389-406.
Summary Statement (from NIH review panel)
RESUME AND SUMMARY OF DISCUSSION: The proposed studies seek to continue the development of a serious game approach for biological discovery. If successful, these studies may bring new insights into predictors of breast cancer prognosis and are therefore viewed as very significant. This submission addresses the majority of concerns raised during the previous review by including a professional game designer and increasing the numbers of players. The panel noted that the investigators are well- qualified but it was thought that a more direct involvement of breast cancer experts would strengthen the team. The environment was judged to be excellent. The panel thought that the use of serious games was a novel approach to discover signatures for breast cancer prognosis. The panel felt that the preliminary results supported the feasibility of the proposed approaches. However, it was pointed out that the prototype results were similar to classifiers developed from just using prior knowledge about cancer genes. This raised the concern that new knowledge may not come from the proposed work. The panel felt that it was unclear how large a population of breast cancer specialists would be needed as players to make the results meaningful. Overall enthusiasm was again mixed among the panel members and, by averaging scores, the proposal is expected to have moderate impact in the fields of bioinformatics and disease.
CRITIQUE 1:
Significance: 2
Investigator(s): 2
Innovation: 1
Approach: 2
Environment: 1
Overall Impact: This proposal aims to create a framework for engaging the larger scientific community to participate in “scientific discovery games” to help generate better predictors of breast cancer prognosis. It is a resubmission of a previous submission. The PIs addressed the key previous critique about the “playability” of the game by engaging a professional game designer and with a demonstration of a large number of participants in the current prototype (>1200). Aim 1 is focused on attracting people to the game and Aim 2 is focused on knowledge capture linking genes to clinical variables.
1. Significance:
Strengths
Improvements in predictors of breast cancer prognosis could certainly have tremendous impact in cancer treatment.
The PIs argue that existing shortcomings in genomic predictors of disease prognosis are due to the lack of a structured knowledge about the disease from which analyses can build from, a challenge that the proposal tackles with the crowdsourcing approach.
Novel methods in developing approaches to crowdsource the development of cancer predictors could have impact in many applications.
Weaknesses
The proposal has a little bit of a mixed focus; it is both an evaluation of how to make a good “game” as well as directly focused on using a good game to identify good genomic predictors of breast cancer prognosis. More clarity to the identity of the proposal would help with plans for its execution.
The proposal makes reference to other scientific gaming activities, but there should be a more direct discussion of what features work and don’t work in those other settings and what about the gaming aspects of this proposal are different, other than simply its focus on building genomic predictors of disease prognosis.
2. Investigator(s):
Strengths
The PI has led many innovative crowdsourcing approaches in biology, including the gene wiki.
The proposal will engage a professional game designer (an “expert on fun”).
Weaknesses
More direct engagement of a breast cancer expert (beyond letters of support) would help guide the team with some critical decisions in the game design.
3. Innovation:
Strengths
Crowdsourcing the generation of predictors of breast cancer prognosis is certainly innovative.
Weaknesses
None noted.
4. Approach:
Strengths
A key critique in the previous submission was the “playability” which was directly addressed with the engagement of a professional game designer.
More than 1200 people have played 10,500 rounds of the prototype game, supportive of the feasibility and quality of the approach.
Strategies to advertise are excellent, with posting to the BioGPS site, engagement of other scientific gaming initiatives, and coupling efforts with MOOCs.
Weaknesses
The crowdsourcing value is a function of the size and the diversity of the group. The proposal does not focus on the “quality” of the participants directly. While there is mention of the “short head” of valuable contributors, this seems like such an important element of the game design and what could be learned that it should be a more direct focus of the work. Perhaps the “experts” contribute much less to the overall knowledge than would be expected. I don’t know if the diversity of backgrounds and scientific training needs to be high or low, but the proposal should evaluate this feature directly.
The proposal discusses great preliminary data on the number of participants, but a key feature would be the number of return participants. How many are coming back? How can you incentivize the return of players?
Structuring the data as decision trees seems appropriate for the game design and a direct way to capture expert knowledge. However, there are assumptions in what is “low” or “high” that should be explicitly explored since such cut-offs can significantly influence results.
There’s no discussion on how a gaming decision is deemed “right” or not. What about a predictor that selects for 60% of the correct diagnoses versus one that is 40% accurate compared to two predictors that are 70% vs. 30% correct?
5. Environment:
Strengths
Resources and environment at TSRI are excellent for the proposed work.
Weaknesses
None noted.

CRITIQUE 2:
Significance: 4
Investigator(s): 1
Innovation: 2
Approach: 4
Environment: 1
Overall Impact: This is an interesting proposal to develop a “serious game” in which user gameplay is used to develop molecular signatures for breast cancer prognosis. The approach has not been used before with this type of bioinformatics data, and it is a somewhat different type of scientific application than the sequence and structure optimization challenges that have been the basis of successful games. Innovation is a strength. I get a much stronger sense of their vision what the game will be like for the second aim, where a concrete and appealing description of how the user will build classifiers and interact with the game is given, than in the first. Significance derives from their ability to attract users, a significant proportion of them experts, and uncertainty about the appeal of the first phase approach and its ability to attract significantly more users than it has so far is a potential concern.

1. Significance:
Strengths
Addresses a clinically significant problem – development of more accurate predictors of breast cancer prognosis from experimental data.
Weaknesses
The project is by nature pretty speculative. A few science games have made a good showing, but in less high-stakes, basic science areas such as protein structure prediction and genome assembly. Can crowdsourced science provide insights that are specific and reliable enough to be used in diagnostics with direct impact on care? The prototype work does not suggest a significant step up over other classifiers.
If the game does not successfully attract users then significance will be greatly reduced – the prototype results were similar to standard classifiers developed based on prior knowledge.
2. Investigator(s):
Strengths
PI and co-I specialize in biomedical data warehousing/data mining and in cognitive science, respectively.
Dedicated funds have been included to support a part-time consultant specializing in game design (Peay) as recommended in the previous review cycle.
Weaknesses
None stated
3. Innovation:
Strengths
Serious games are becoming more common, but this is still a fairly novel approach to development of disease classifiers based on molecular data.
Weaknesses
None stated
4. Approach:
Strengths
In general, the gameification approach is popular and I have no trouble believing they will find a base of participants. Undergraduate bioinformatics students love Foldit.
The decision tree building tool that they propose to develop in Specific Aim 2 – allowing users to build a hypothesis in the form of a decision tree classifier and then validate it (or not) using the aggregated information in the database seems like it would be a fairly useful interactive data mining tool for researchers trying to integrate large volumes of data and the kind of tool that could be useful in a non-game environment. It should incentivize experts to participate and to contribute data.
Weaknesses
Preliminary results with a limited set of users in a prototype game resulted in a classifier that performed very similarly to classifiers just using prior information about cancer genes. Isn’t a general population with access to and experience of, perhaps, college level textbooks in the life sciences, going to produce a “crowd wisdom” that is centered around exactly such prior knowledge?
Despite creating a mechanism for non-expert users to participate through a leveling system, the game still relies heavily on qualified specialist participants to generate valuable insights.
The proposal doesn’t give me a good feel for what the first phase game mechanics will be like– what comes after the prototype but before the decision tree building? That experience isn’t as well described. That step seems to be critical to engaging users initially.
5. Environment:
Strengths
They have an enthusiastic group of supporters (see letters) who are willing to participate in the development of expert-level classifiers which is key to the second specific aim.
They have identified a group of scientific game developers who are willing to cross-promote The Cure to a community of users who have already demonstrated interest in scientific games.
The host institution is well equipped to sustain the proposed project.
Weaknesses
None stated
CRITIQUE 3:
Significance: 3
Investigator(s): 3
Innovation: 4
Approach: 4
Environment: 3
Overall Impact: The authors propose to improve prediction in breast cancer based of clinical genomics data through a crowdsourcing approach of serious game playing. The approach to solving the problem is interesting and the investigators appear to be a “dream-team” for this project. My concern is that this may be only an interesting exercise on how collective mind works. Of course, if it is effective (and the gained knowledge is translatable to a method or algorithm), it would be of great importance to the community.
1. Significance:
Strengths
The problem of better prediction in breast cancer is an important problem.
Crowdsourcing is becoming an interesting tool for tricky, ill-understood problems in general.
Weaknesses
None.
2. Investigator(s):
Strengths
The investigators are best suited for this project, with background in breast cancer and designing games for crowdsourcing.
Weaknesses
None.
3. Innovation:
Strengths
The approach of using serious games to improve prediction is very interesting.
Weaknesses
None.
4. Approach:
Strengths
The preliminary data with the currently implementation has already attracted many players.
The strategy and game plan appears sound as demonstrated by the authors in their earlier work.
Weaknesses
How large is the population of specialized players (breast cancer biologists) to make this game meaningful?
5. Environment:
Strengths
The host institutes are well-suited.
Weaknesses
None.
Here are the summaries of the scores for apps 1 and 2 for comparison.
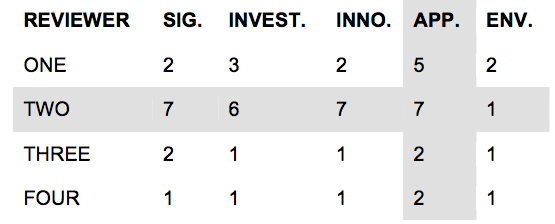 |
Scores from original submission (9-point rating scale (1 = exceptional; 9 = poor) ) Impact score = 55
|
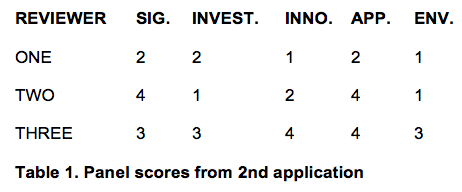 |
Impact score = 40 |
Having read this I thought it was extremely informative. I appreciate you taking the time and effort to put this article together.
I once again find myself personally spending a significant amount of time both reading and commenting.
But so what, it was still worthwhile!